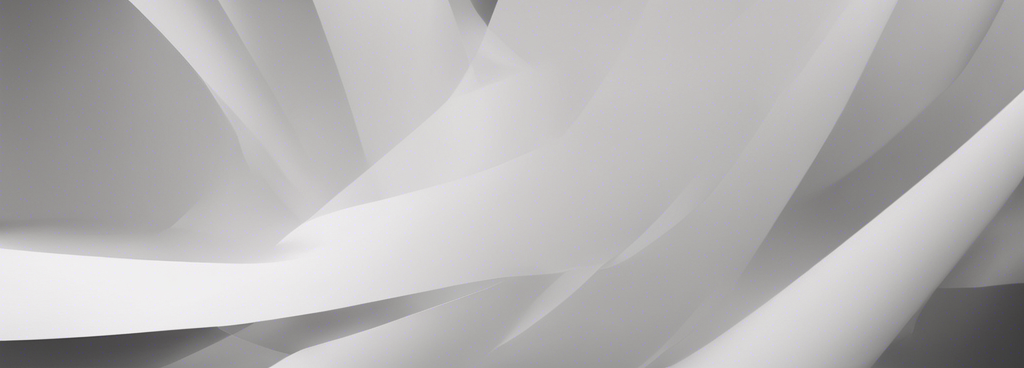
Optical Character Recognition of Low-Resolution Text
The team at MCQ-Scan, presents a novel method for the detection and recognition of low-resolution text in real-time.
Overcoming the Challenges of Low-Resolution OCR in Real-Time
The task of accurately detecting and recognizing text in low-resolution images is fraught with challenges, from varying illumination levels and contrast to blurring and geometric distortions. These issues are particularly pronounced in the retail environment, where inventory robots must decipher price tags under less-than-ideal conditions.
Traditional OCR methods, which typically employ separate neural networks for detection and recognition, fall short in terms of speed and accuracy when faced with such low-resolution text.
Our method stands out in the competitive landscape, offering an exceptional recognition rate at a significantly reduced inference cost.
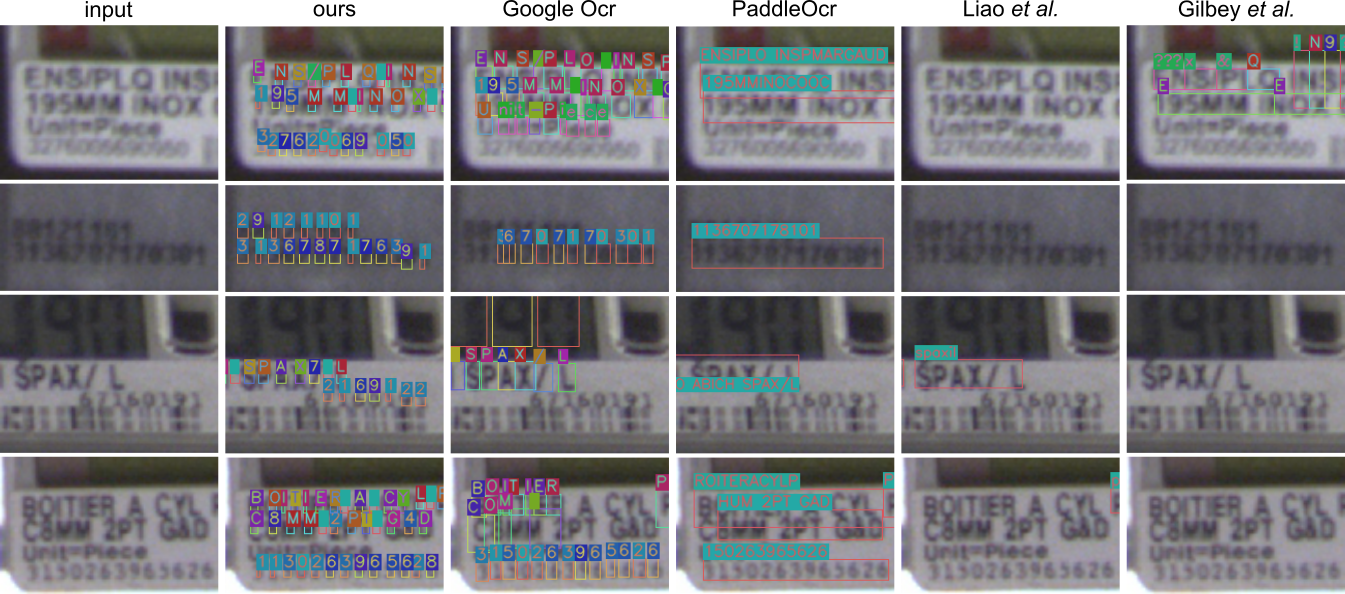
A Two-Pronged Approach to Precision and Speed
The MCQ-Scan team’s research introduces a two-fold solution to this problem. Firstly, we have developed a pipeline for generating synthetic datasets tailored for low-resolution text, significantly reducing the need for manual annotation. Secondly, we utilize a streamlined neural network architecture based on the U-Net model, capable of performing text detection and recognition simultaneously. This innovative approach not only achieves real-time processing speeds of over 120 frames per second but also maintains high accuracy even when characters are as small as five pixels in width.
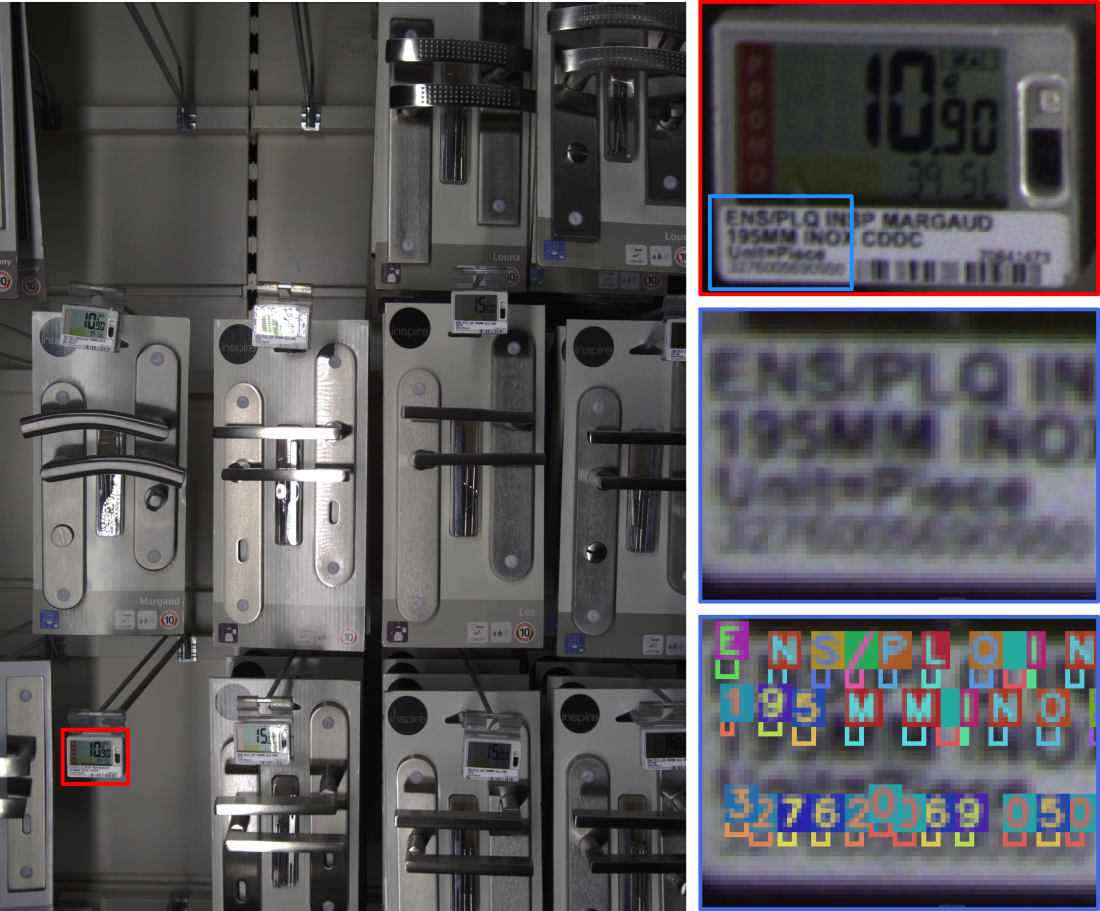
Real-World Application and Adaptability
The implications of this research are vast, offering a highly adaptable solution that can be fine-tuned for a wide array of applications beyond retail inventory management. The method’s superior performance in recognizing low-resolution text under challenging conditions demonstrates its potential to revolutionize OCR technology.
This demo showcases an OCR solution tailored for robots that navigate and photograph product display aisles. Since the primary focus isn’t on pricetags, they may appear small and out of focus, our method can still extracting crucial information from them. Explore the functionality by using the provided sample pricetags captured by our robot, or upload and crop your own photos to focus on the pricetag.